- Nextant
- 6 days ago
- 5 min read
You Have the Data. Why Aren't You Ready for AI?
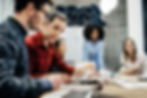
Rapid-growth companies often believe that having vast amounts of data means they're AI-ready. Unfortunately, that's a major misconception. Disorganized systems and old tools can hurt growth. Undocumented processes and scattered data make it hard for AI initiatives to begin. They can stall before they even start.
Today, data-rich companies face clear AI challenges: what got you here, won’t get you there. To use AI for smarter, faster growth, we must first focus on data quality and operational maturity. Without these, it remains just a dream.
Common Misconceptions About AI Readiness
Many companies confuse "having data" with "being ready for AI." But success with AI depends less on the amount of data and more on the state of it. Without complete and reliable data, AI models can give wrong results. This can mislead businesses and take them off track.
(For deeper background on this topic, see The Importance of AI Readiness)
The Hidden Costs of Disorganized or Siloed Data
Ignoring your data issues doesn't just delay AI. It actively drains the business:
Slower Decision-Making: Teams spend valuable hours reconciling inconsistent reports.
Missed Automation Opportunities: Disconnected systems prevent process automation.
Decreased Trust: Leaders rely on gut instinct because data can't be trusted.
Scaling Friction: Systems break under pressure, creating ceilings on growth.
Failed AI Initiatives: AI tools fed by bad data produce bad insights — at high costs.
“Outdated or conflicting information quietly drains time and money from a company. These problems are hidden, but they add up and slow down the whole business over time.” — Sebastian Sanchez, Head of AI.
“Without trustworthy, unified data, even the best strategies will fail.” — Enrique Monsalve, Head of Business Process Modernization
Why "What Got You Here Won't Get You There"
At the "critical scaling phase," companies face an inflection point. Startup-style improvisation that once enabled growth now backfires. Data becomes fragmented across departments, systems don't scale, and manual processes start collapsing under volume.
If you wait until this dysfunction becomes a crisis, you're already behind.
“Companies that fail to evolve their systems during scaling phases typically stall when team and system overwhelm occurs. To survive and scale, you must go beyond 'hero employees' and set up sustainable systems that can survive specific employees.” — Ernesto Samudio, Head of Products and Services
Rapid Growth = Hidden Data Problems
Fast-growing companies often:
Collect massive volumes of operational and customer data.
Lack unified systems to organize, cleanse, or connect that data.
Build department-specific tech stacks with little cross-team integration.
You have the data; but if it’s messy, siloed, and undocumented, it can’t be trusted or easily leveraged.
(For more on this, see Understanding Data Maturity: Where Does Your Business Stand?)
Foundational AI Adoption Challenges Every Business Must Solve
AI is becoming key in business strategy. Leaders now face fresh challenges with AI adoption. Many organizations struggle with complex data, limited skills, and unclear processes. These issues create major roadblocks. What are the main challenges in implementing AI solutions? Common issues are poor data quality, broken systems, and unrealistic expectations about AI. If companies ignore these key issues, they risk failed deployments, wasted resources, and missed opportunities. This shows some of the toughest AI challenges in business today.
Generative AI Challenges and the Roadblocks to Successful Implementation
Generative AI brings great potential for innovation. But it also comes with new risks.
Key challenges in generative AI are:
Data privacy
Output reliability
Integration with legacy systems
Generative AI adds to the wider challenges that businesses face when implementing AI. Companies using AI face technical challenges and business consulting issues. These include cultural resistance and strategic misalignment. To tackle these AI industry challenges, you need solid data governance. You also need clear leadership and a step-by-step deployment plan.
Where AI Fits In — and Where It Doesn't (Yet)
Jumping directly to Generative AI without fixing foundational issues is a recipe for failure. AI can create great value, but only if your data is organized. This is a key reason for many AI challenges faced by data-rich companies today.
The most opportune early wins for a rapidly scaling company include:
Create Strong Dashboards: Combine your key KPIs and operational data into one reliable source. These dashboards need a unified software system. This ensures data accuracy and consistency.
Use AI agents. They help employees find information fast. They can do calculations and get insights from dashboards and reports. AI agents can automate tasks. They can summarize reports, find key metrics, and flag inconsistencies.
Using Predictive Analytics: After creating solid data systems, AI can predict trends. It can also find potential problems early and enhance resource planning.
Without this solid base, AI efforts will be ineffective, costly, and potentially harmful.
“The potential of AI lies in generating meaningful insights. But if your information isn’t unified, AI will not work as intended, and costs will skyrocket.” — Natalia Silva, Head of Data and Analytics
Urgency: Act Now or Pay Later
If you delay:
Growth slows as complexity eats your momentum.
Manual inefficiencies breed tech debt.
Future projects, such as AI or automation, increase in difficulty and cost at an exponential rate.
Leadership loses trust in data, making strategy slower and more reactive.
If you act now:
Faster, smarter decisions drive competitive advantage.
Foundation is ready for scalable AI and automation.
Margins improve as operations become leaner and more data driven.
The company avoids "growth ceiling" traps that kill momentum.
Recent Case Studies: How Good Data and AI Agents Drive Success
Super.com, a fast-growing travel-fintech platform, partnered with Glean to improve data management and accelerate decision-making across its remote workforce. Using Glean’s AI-powered enterprise search tool, Super.com centralized information from Slack, Confluence, and Google Drive, making it instantly accessible based on user roles.
Key Results:
Saved over 1,500 employee hours per month.
Cut new hire onboarding time by 20%.
Introduced AI tools to help prioritize tasks and draft communications.
Super.com is now expanding into AI agents capable of autonomously handling tasks like meeting scheduling.
Johnson Controls expanded its OpenBlue Enterprise Manager suite by integrating advanced AI capabilities through Microsoft Azure and Power Platform. This initiative introduced customer-facing generative AI applications and more autonomous building controls.
Key Outcomes:
Achieved up to 30% reduction in energy expenditure.
Realized up to 20% decrease in maintenance costs.
Optimized space utilization by 10%.
This case highlights how a unified software ecosystem and AI agents can drive significant operational efficiency and faster decision-making.
How to Start Now
Audit your data: Where are your inconsistencies, silos, duplications? Is your taxonomy adequate? Are your data labels self-explanatory?
Prioritize integration: Consolidate core systems and processes first. You have several options here, integrating systems or building data repositories are the most common strategies.
Document your data models: establish clear standards and definitions.
Focus on quick wins: Create easy dashboards and use AI agents to boost data access.
Plan for scale: Pick systems and structures that can handle 10x growth. This will set you up for predictive analytics.
Final Thought
Companies that organize and unify their data early don’t survive. They grow quickly, innovate efficiently, and leave slower competitors behind.
The window to fix data before AI expectations explode even higher is closing.
Move now — or you will have to catch up later at 10 times the cost.